FURI | Fall 2021
A Systematic Study on Quantifying Bias in GAN-Augmented Data
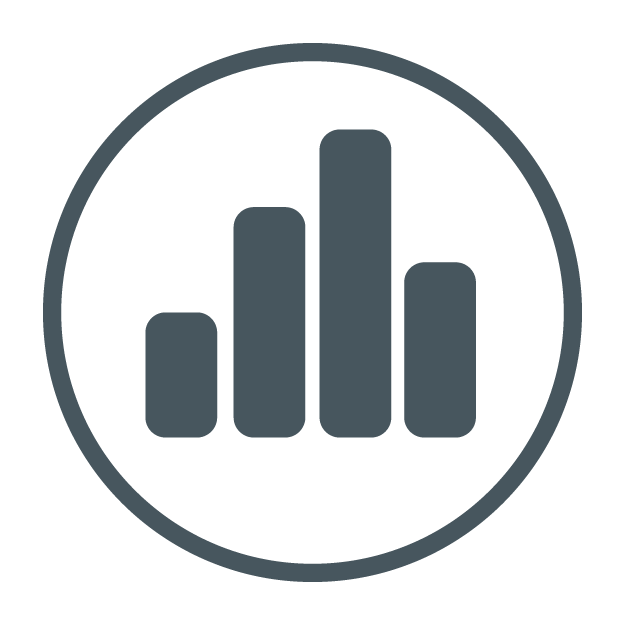
Generative Adversarial Networks (GANs) can be used to augment the amount of information in datasets. However, upon training on certain datasets, GANs can magnify already existing biases in them. In image datasets, biases pertaining to protected attributes such as race or gender could be exacerbated, resulting in harmful societal consequences. This study aims to evaluate a series of quantitative measures that best measure the extent to which biases are exacerbated in GAN-augmented datasets and indicate whether adjustments should be made before using GANs as an augmentation method.
Student researcher
Denis Feiduo Liu
Computer science
Hometown: Tempe, Arizona, United States
Graduation date: Spring 2023