FURI | Fall 2023
Resolution-Invariant Image Classification via Implicit Neural Representations: An Application to Medical Whole-Slide Imaging
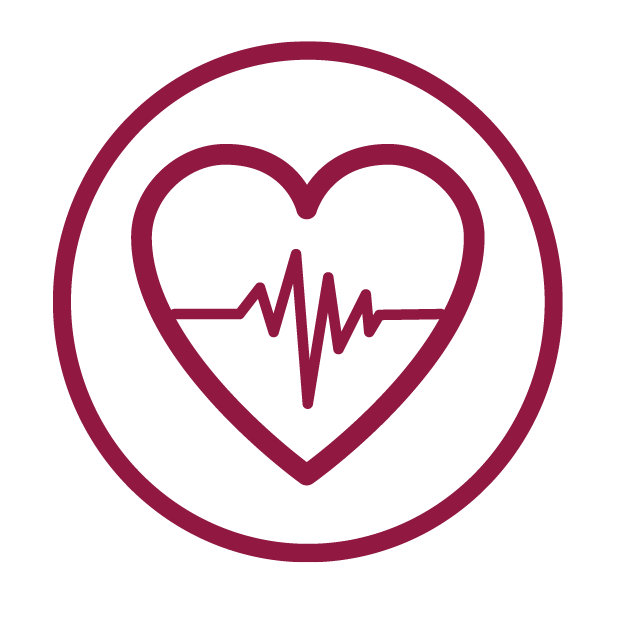
Deep Neural Networks (DNNs) have achieved remarkable success in image recognition and classification. However, prevailing network architectures demonstrate constraints regarding the input resolution they can process effectively. This study introduces an approach that leverages implicit neural representations extracted from images at varying resolutions as input to neural networks. Such a methodology is particularly advantageous for classifying medical images, notably in the context of whole-slide imaging. These images often contain giga-pixel resolutions, complicating conventional classification efforts. This research offers a potential path for more memory-efficient classification tasks on vast medical images.