FURI | Fall 2024
Building YMS (Yard Management System) using Machine Learning and Optical Character Recognition Technology
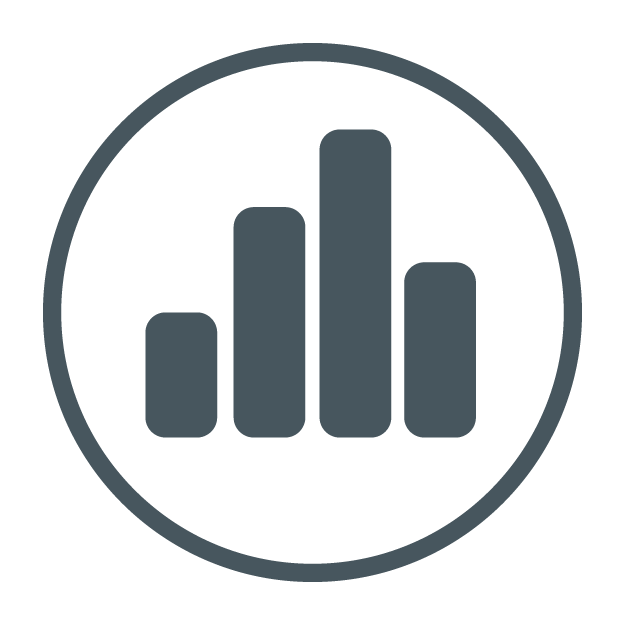
This project addresses the research question: “How can emerging technologies such as Machine Learning (ML), Optical Character Recognition (OCR), and advanced computer vision frameworks like YOLOv10 be leveraged to optimize yard management processes and reduce inefficiencies in logistics operations?” By integrating AWS Textract for accurate OCR processing and YOLOv10 for real-time object detection from CCTV footage, the project aims to automate the identification and processing of container numbers, vehicle details, and driver information at entry gates. This transition from a manual system to an automated one addresses common issues such as human error and gate congestion during peak periods, ultimately reducing processing times and improving data accuracy. However, as these technologies advance and become more integrated into operational workflows, they raise ethical concerns about the displacement of human labor. While automation may create a more comfortable work environment by alleviating tedious tasks, it also invites skepticism regarding its potential to replace jobs traditionally held by humans in logistics and transportation. This project not only seeks to enhance operational efficiency but also encourages reflection on the broader societal implications of AI and ML in the workforce.